Current Projects
"NSF CAREER: Distributionally Robust Learning, Control, and Benefits Analysis of Information Sharing for Connected Autonomous Vehicles." , funded by NSF.
“SCC-IRG Track 1: Socially Informed Services Conflict Governance through Specification, Detection, Resolution and Prevention.” , funded by NSF.
"CPS: Small: COLLAB: Improving Efficiency of Electric Vehicle Fleets: A Data-Driven Control Framework for Heterogeneous Mobile CPS", funded by NSF.
"S&AS: FND: COLLAB: Adaptive and Cognizant Vehicular Sensing and Control for Fleet-Oriented Systems in Smart Cities", funded by NSF.
“Robust Control Protocol Synthesis and Safe Learning for Connected Autonomous Vehicles ”, May 2019-June 2020, funded by Uconn Research Excellence Program (REP).
"S&CC: Empowering Smart and Connected Communities through Programmable Community Microgrids", funded by NSF.
"Modeling, Analysis and Anomaly Detection for Cyber Secure Eversource Power Distribution Networks", funded by Eversource Energy, a northeast energy company.
"Energy Management Systems for Subtractive and Additive Precision Manufacturing" , funded by Clean Energy Smart Manufacturing Innovation Institute.
Safe and Robust MARL and Control for Networked CPS
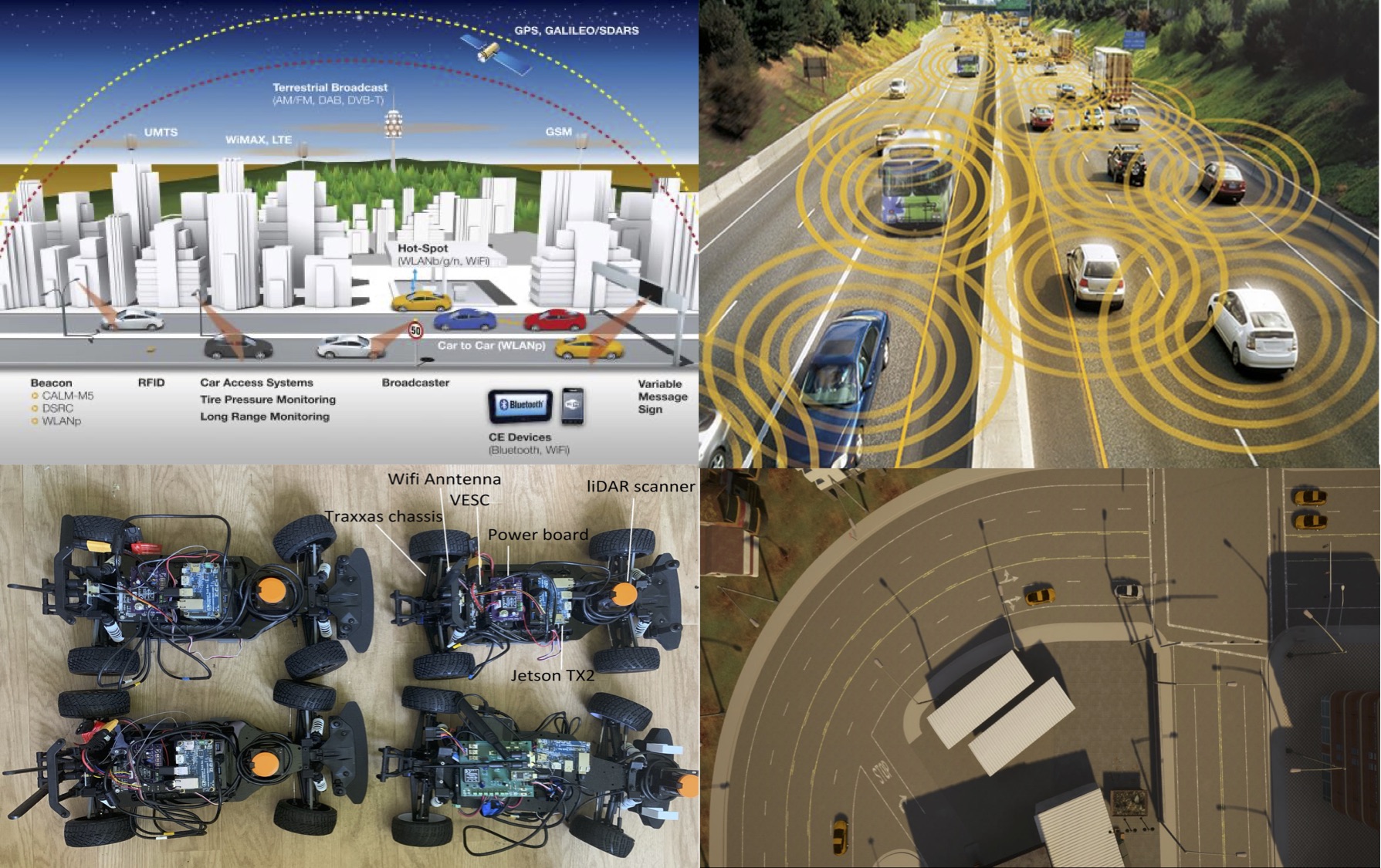
Detail of the project and related publications can be found through the project webpage. Vehicle-to-vehicle (V2V) and Vehicle-to-Infrastructure (V2I) wireless connectivity is the next frontier in road transportation, which will greatly benefit the safety and reliability of autonomous cars. Information shared among autonomous vehicles provides opportunities to better coordination schemes and also raises novel challenges. In the future, connected autonomous vehicles (CAVs) equipped with both self-driving technology and V2V connectivity, will lead to vastly improved road safety and efficient traffic flow. However, existing literature for connected vehicles or platoons still lacks understanding of the tridirectional relationship among communication, learning, and control of CAVs. Questions such as under what conditions coordination among vehicles can be built, or how to take the best advantage of shared information to improve safety of the connected vehicles and efficiency of the traffic flow remain challenging. Hence, it is critical for future connected autonomous vehicles systems to operate based on integrated learning and control theories, techniques and coordination protocols under complicated environments. This project aims to build fundamental theories and implement experiments for a safe and efficient decision-making process of autonomous vehicles under dynamic and uncertain environment. The benefits of sharing different types of information is analyzed at different scales, from system level efficiency to safety guarantee of each individual autonomous vehicle. Experiments will be implemented with both 1/10th scale racing cars and full-scale autonomous vehicles on Uconn Depot Campus by collaborating with the Connecticut Transportation Safety Research Center.
In Summer 2021, I hosted a virtual research site for two minority scholars of the Young Scholars Senior Summit (YSSS) based on research activities of this award. See more information in Outreach.
"NSF CAREER: Distributionally Robust Learning, Control, and Benefits Analysis of Information Sharing for Connected Autonomous Vehicles" , funded by NSF.
“Robust Control Protocol Synthesis and Safe Learning for Connected Autonomous Vehicles ”, May 2019-June 2020, funded by Uconn Research Excellence Program (REP).
Relevant publications: [SafeMARL_ICRA'23] [UQPerception_ICRA'23] [ICRA'22] [StateAdversarial_MARL_arXiv22] [SafeMARL_Journal22] [CDC'19]
MARL and Robust Optimization for Sustainable Mobile CPS
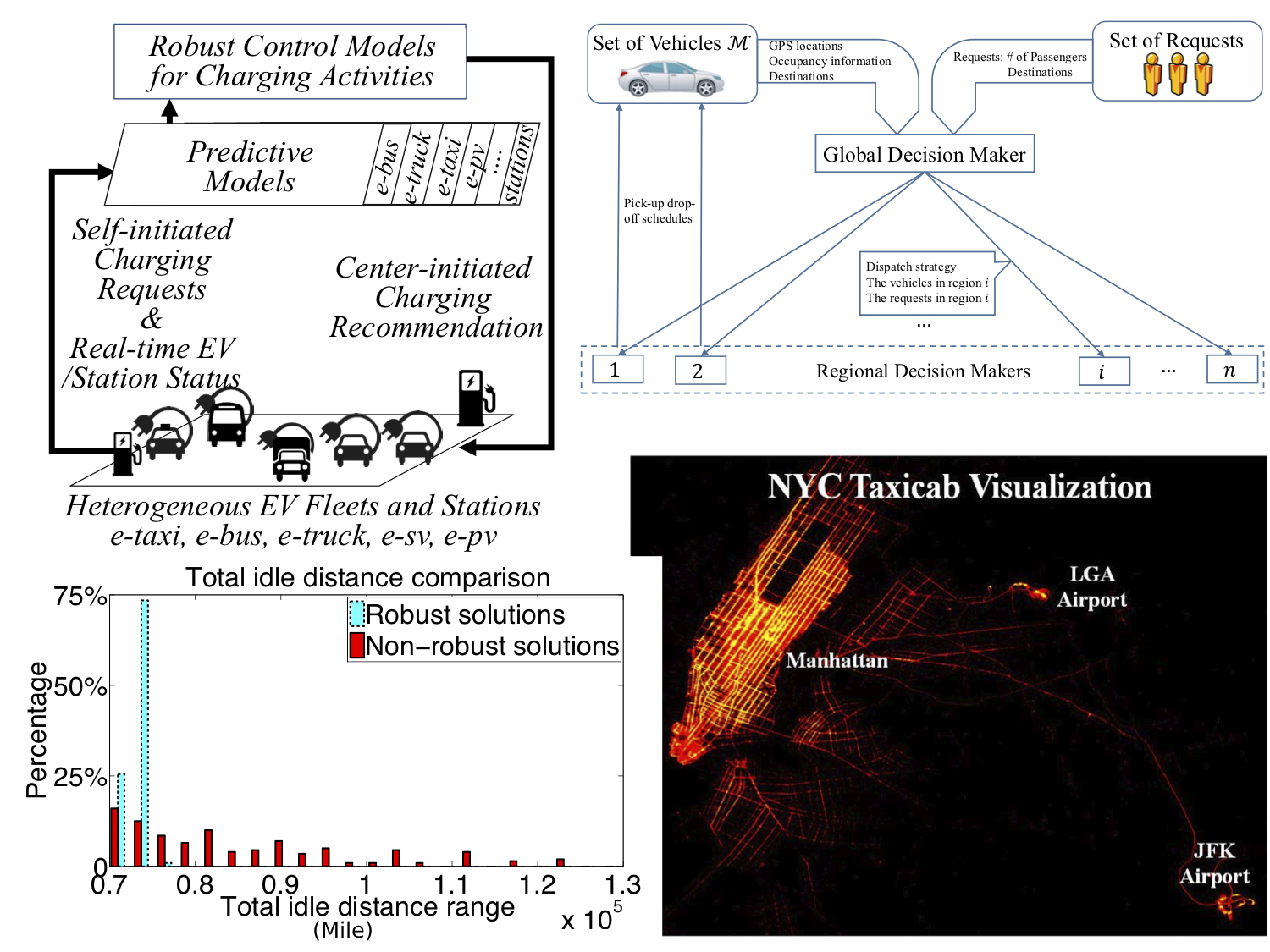
Detail of the project and related publications can be found through the project webpage. Ubiquitous sensing in smart cities enables large-scale multi-source data collected in real-time, poses several challenges and requires a paradigm-shift to data-driven cyber-physical systems (CPSs) that integrates optimization, control and machine learning. For instance, how to capture the complexity and analyze the dynamic interplay between urban-scale phenomena from data, and take actions to improve service efficiency and safety, is still a challenging problem in transportation systems. My work about real-time robust resource allocation problem in smart cities falls in the intersection of machine learning, optimization and control. We design a data-driven dynamic robust optimization framework for autonomous ride-sharing and carpool systems, matching vehicle supply towards both current and predicted future demand. With spatial-temporal uncertainty of demand prediction, we prove and develop computationally tractable methods that provide probabilistic guarantees for the system’s worst-case and expected performance. Dynamic pricing and hierarchical carpool algorithms are also designed for travel time reliability during peak hours. We show that the performance of the ride-sharing system is improved based on world taxi operational data. For electric vehicles such as E-buses and E-taxis, we consider both the mobility pattern and charging pattern to co-design the charging and passenger pick-up algorithms. Heterogeneous data, uncertainties of the dynamic status of the EV system, tradeoff among objectives and benefits of different components of the system are the main focus for current work.
"CPS: Small: COLLAB: Improving Efficiency of Electric Vehicle Fleets: A Data-Driven Control Framework for Heterogeneous Mobile CPS", funded by NSF.
Relevant publications: [IEEE_TITS23] [TCPS20] [IROS'20] [ICCPS'18] [CDC'17] [TCST19][ICCPS'17][TASE16][CDC'15][ICCPS'15]
Adaptive Vehicular Sensing and Control for Fleet-Oriented Systems
In smart cities of the future, how to sense, understand, and manage urban-scale vehicular systems, e.g., taxis, in an autonomous fashion (with little or no human intervention) is an essential topic to improve urban mobility efficiency, such as shorter waiting time for passengers, lower cruising mileage for drivers, and higher revenues for vehicular system operators. However, the current management strategies for vehicular systems are mainly based on individual-level data knowledge, ignoring rich information from a fleet perspective. This research develops a fleet-oriented sensing and control framework to enable seamlessly integration of historical and real-time data within a fleet for adaptive vehicular sensing, modeling, and control. Specifically, this project studies how to best use spatiotemporally-correlated contextual information (e.g., vehicular mobility, service demand, disruptive events) among vehicles. Although such correlations decay over time and distance, it is possible to enable autonomous vehicular sensing, modeling, and control adaptively based on the following research to develop novel services: (i) reconfigurable fleet-wide coordinated sensing by autonomously learning correlated vehicular interactions; (ii) models of mobility phenomena by collectively interpreting implicit data from different vehicles with a combination of deep learning, structured learning, and attribute-based learning; (iii) designs of robust dispatching strategies with uncertainty sets and receding horizon control frameworks by iteratively considering fleet-wide knowledge to improve mobility efficiency.
"S&AS: FND: COLLAB: Adaptive and Cognizant Vehicular Sensing and Control for Fleet-Oriented Systems in Smart Cities", funded by NSF.
Relevant publications: [TCPS20] [IROS'20] [CDC'19]
Attack Detection and Resilient Control of Cyber-Physical Systems
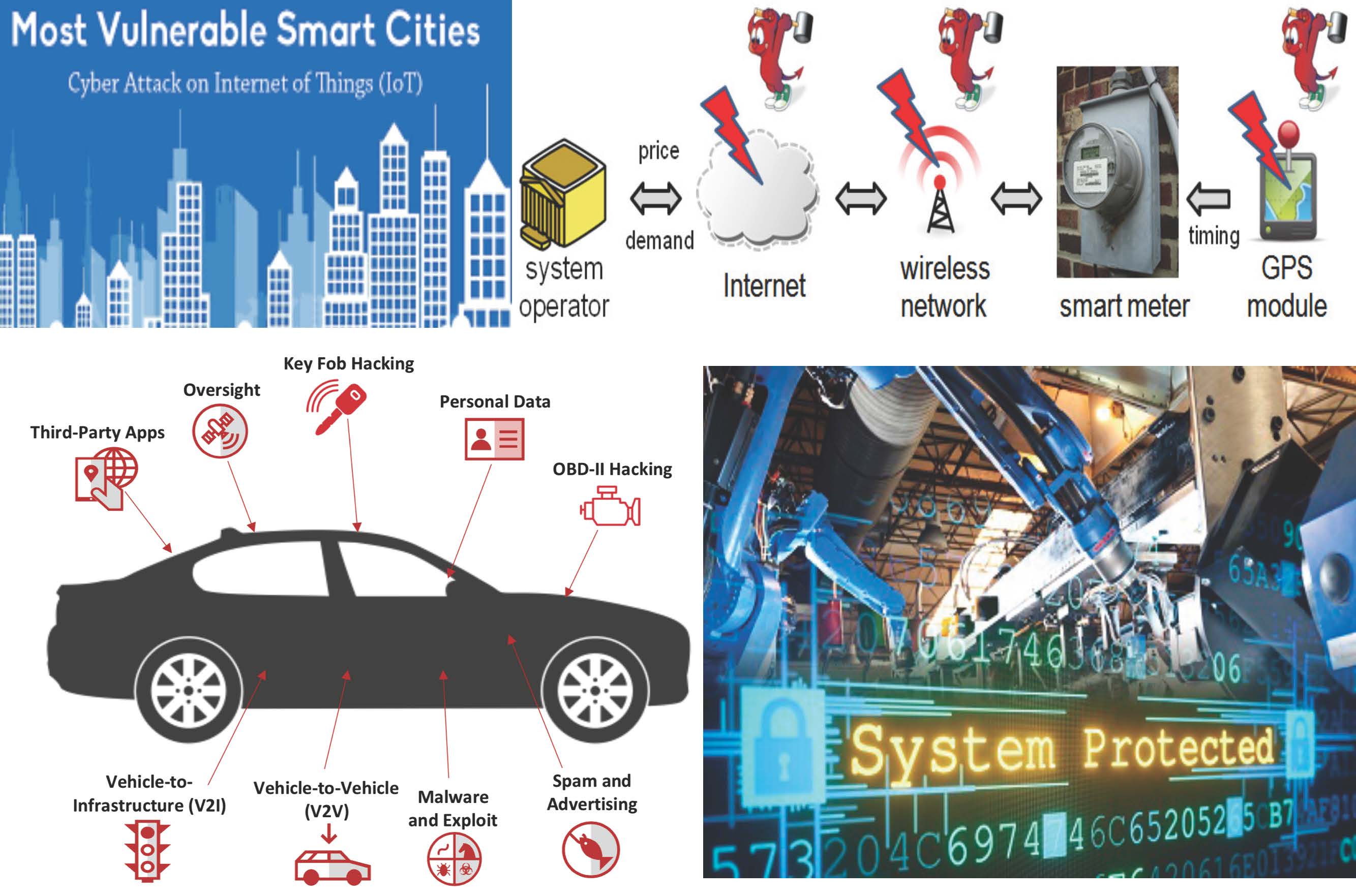
Cyber-physical systems form a ubiquitous, networked, computing substrate that underlies much of modern technological society, such as autonomous vehicles and intelligent transportation systems, power network and smart grids, smart manufacturing systems. Researchers have shown that these kinds of networked embedded systems are vulnerable to remote security attacks, and such attacks can cause physical damage while hiding the effects from system state monitors or controllers. The requirement of designing secure networked control systems introduces new challenges. It is necessary to design attack resilient control schemes and architectures, capable of dealing with attacks on the environment of the controller, including attacks on sensors, actuators, and communication media. For a general linear system model with networked sensors and actuators, we design a hybrid state, finite horizon, zero-sum, stochastic game approach to obtain an optimal control policy that balances the security overhead with control cost. The controller switches between subsystems in presence of different types of attacks. We also design time-varying coding techniques with respect to sensor outputs for detecting stealthy data injection attacks to the communication channels. For power system, we analyze the effects of power botnet attacks on distribution network, and design learning based attack detection and localization algorithms.
"Modeling, Analysis and Anomaly Detection for Cyber Secure Eversource Power Distribution Networks", funded by Eversource Energy, a northeast energy company.
"Energy Management Systems for Subtractive and Additive Precision Manufacturing" , funded by Clean Energy Smart Manufacturing Innovation Institute.
Relevant publications: [Automatica18] [TCNS16] [CDC'14] [CDC'14] CDC'13]
Game Theoretic Security Framework for Quantum Cryptography
We analyze quantum key distribution (QKD) protocols through a game theoretic framework. We make several contributions in this work. We propose a general-purpose framework allowing for a game-theoretic analysis of QKD protocols. Our method is the first such framework to allow for critical security computations, as mentioned, and we demonstrate this on several protocols and attack scenarios. Furthermore, the method proposed in this work requires fewer assumptions made on the part of the honest users, which makes it more useful. We show how our method can be applied to practical, real-world devices (which, to our knowledge, has never been considered in any previous game-theoretic approaches). Finally, we make several interesting observations about the efficiency and noise tolerances of QKD protocols operating against rational adversaries. In particular, we show that, under natural assumptions, if an adversary is rational, as opposed to simply malicious, users may increase QKD key generation rates beyond what is possible in the standard adversarial model. Unlike other game theoretic models, we show how important key-rate and noise tolerance computations may be performed through our system allowing for interesting comparisons to the “standard adversarial model” of QKD. We show that, depending on the relative cost of operating devices, increased noise tolerance and improved secure communication rates are possible if one assumes adversaries are rational as opposed to being malicious.
Relevant publications: [Quantum Infor Process'20] [GameSec'18]
Wireless Control Network and Wireless Sensor Network
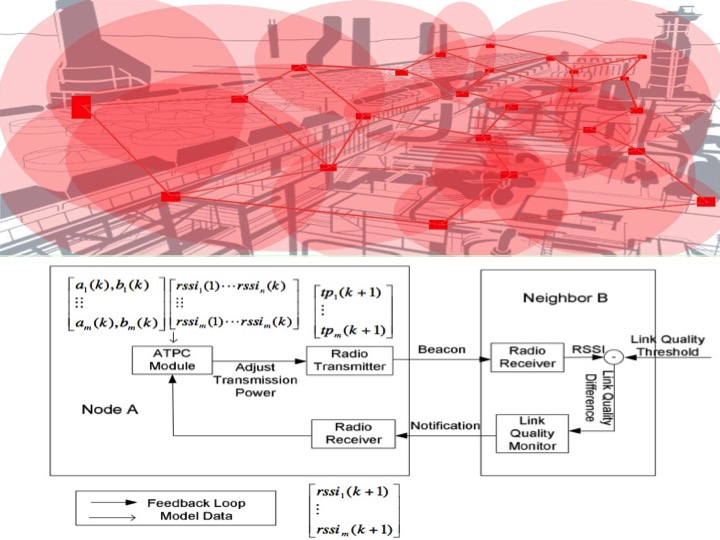
Wireless Control Network (WCN) is a network architecture where the network itself behaves as a distributed, structured dynamical compensator. We study the problem of mapping discrete-time linear controllers into potentially higher order linear controllers with predefined structural constraints. The quality of radio communication between low-power sensor devices varies significantly with time and environment. This phenomenon indicates that the previous topology control solutions, which use static transmission power, transmission range, and link quality, might not be effective in the physical world. To address this issue, we design ATPC, a lightweight feedback-based algorithm for Adaptive Transmission Power Control, an online transmission power control that adapts to external changes. In ATPC, each node builds a model for each of its neighbors, describing the correlation between transmission power and link quality. The results from the real-world experiments demonstrate more energy savings with a finer tuning capability, and robustness even with environmental changes over time.
Relevant publications:
[ACC'13]
[TOSN16]